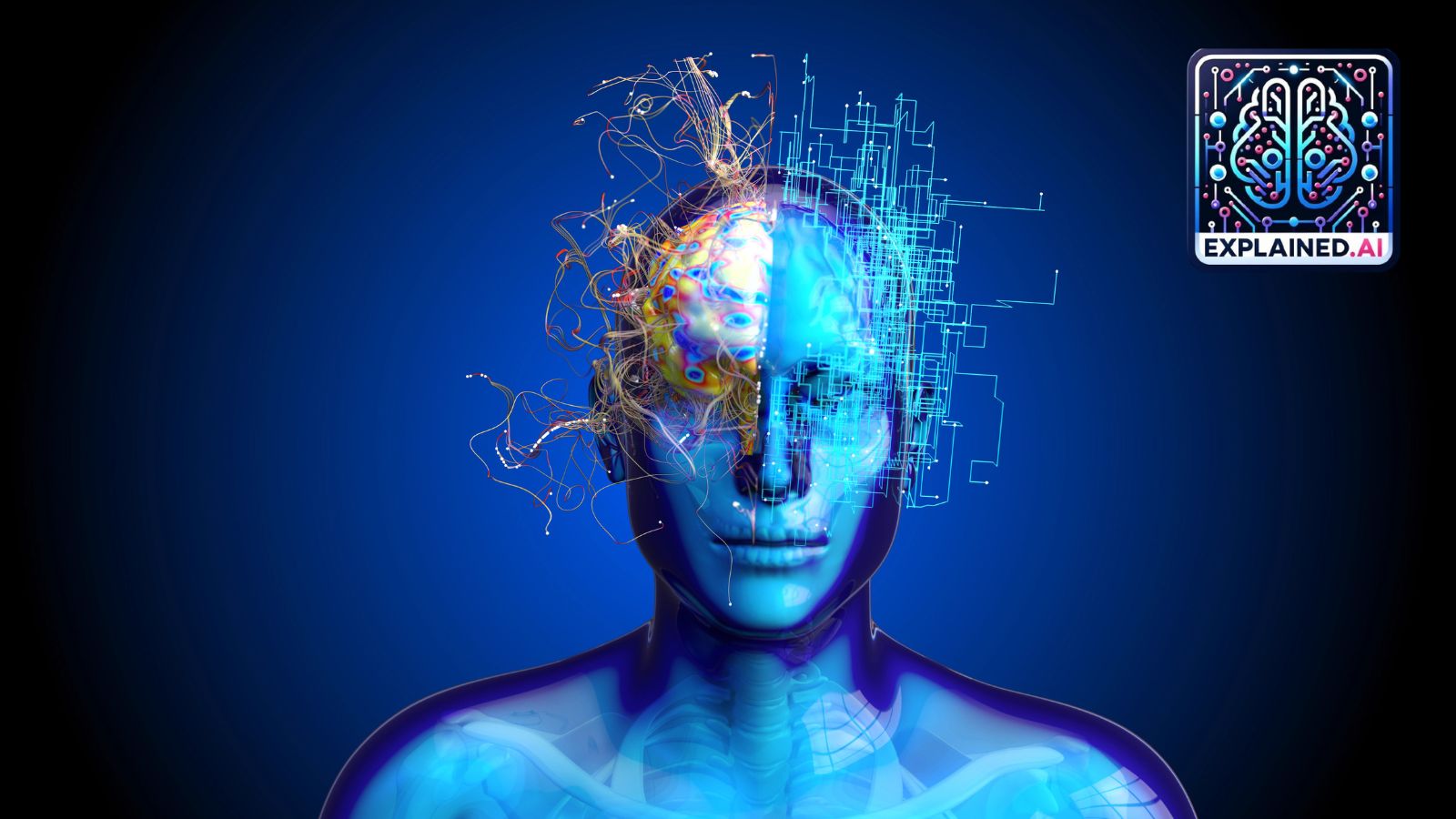
[ad_1]
Almost every time there is progress in the world of Artificial Intelligence (AI), there is discussion on Artificial General Intelligence (AGI), which is broadly defined as AI that can match human intelligence in various tasks.
Tech and AI companies keep proposing timelines of when the world can reach AGI, and it is a term that we recur more frequently as AI models get better.
In a paper published earlier this month, researchers from DeepMind, Google’s AI lab, said it was “plausible” that “powerful AI systems will be developed by 2030”.
But this could cause “severe harm”, the paper said, including “incidents consequential enough to significantly harm humanity”. The paper, published on April 2, proposed a framework for technical AGI safety and security.
But what exactly is an “intelligent” machine, what is it supposed to do, and how will one be built? These questions have occupied the minds of scientists, philosophers, science fiction writers and fans for decades.
This is the story of AGI and “intelligent” machines.
The fascination with — and concern about — intelligent machines is at least three-quarters of a century old.
In 1950, the British mathematician and father of theoretical computer science, Alan Turing, asked: “Can machines think?”
To find out, Turing proposed a test. If a machine could replicate language and communicate to a level where a human could not tell it was a machine, then it could be considered intelligent.
Story continues below this ad
Six years later, a meeting was organised at Dartmouth by John McCarthy, then a mathematics professor at the university, “to proceed on the basis of the conjecture that every aspect of learning or any other feature of intelligence can in principle be so precisely described that a machine can be made to simulate it”.
This, more or less, laid the foundation of the field of AI.
In 1970, computer scientist Marvin Minsky, who taught for a long time at Massachusetts Institute of Technology (MIT) and set up the institute’s AI lab, predicted that in three to eight years, there would be “a machine with the general intelligence of an average human being” — one that would be “able to read Shakespeare, grease a car, play office politics, tell a joke, have a fight”.
This machine, Minsky said, “will begin to educate itself with fantastic speed”, “in a few months it will be at genius level, and a few months after that, its powers will be incalculable”.
Story continues below this ad
Things did not progress quite that fast. But AI researchers continued to work on McCarthy’s and Minsky’s ideas, experimenting with various ways to build “intelligent” machines.
Some of the ideas and concepts that were developed along the way form the basis of how machine learning algorithms — which power today’s AI from your Netflix recommendations to Large Language Models (LLMs) such as ChatGPT, Bard, and Claude — work.
But it was not until the 1990s that the term AGI came to be used widely.
An American physicist called Mark Gubrud is believed to have first used and defined AGI in a paper on military technologies published in 1997.
Story continues below this ad
AGI, Gubrud said, meant “AI systems that rival or surpass the human brain in complexity and speed, that can acquire, manipulate and reason with general knowledge and that are usable in essentially any phase of industrial or military operations where a human intelligence would otherwise be needed.”
In 2001, Shane Legg, who is now the chief AGI scientist at Google Deepmind, suggested to his friend, the computer scientist Ben Goertzel, that a book of essays about AI that he was co-editing at the time, should be called ‘Artificial General Intelligence’.
“I was talking to Ben and I was like, ‘Well, if it’s about the generality that AI systems don’t yet have, we should just call it Artificial General Intelligence… And AGI kind of has a ring to it as an acronym,” Legg is quoted in a 2020 article in the MIT Tech Review.
By generality, Goertzel meant an idea in which AI systems could do multiple things — in the same way as a human can do math, swim, write, think, research, interact, and so on, all of which encompass what would be broadly called ‘intelligence’.
Story continues below this ad
Artificial General Intelligence, eds. Goertzel and Cassio Pennachin, was published in 2007. The following year, the Conference on Artificial General Intelligence (AGI), an annual meeting of researchers in the field, was launched.
For a lot of these people, the stated goal for the AI world was now defined: achieving AGI. It became acceptable and challenging to figure out ways to achieve what some had earlier dismissed as wishful thinking.
But still, what did the term AGI really mean?
There isn’t a universally accepted definition yet.
Goertzel and Legg kept it broad: the ability to do “human cognitive tasks”.
More than a decade later, Murray Shanahan, a professor of cognitive robotics at Imperial College London and a scientist at DeepMind, defined AGI as “artificial intelligence that is not specialized to carry out specific tasks, but can learn to perform as broad a range of tasks as a human.” (The Technological Singularity, 2015)
Story continues below this ad
Shanahan included learning tasks as a factor in defining AGI.
In December 2015, OpenAI Inc., the company behind ChatGPT, was founded to develop “safe and beneficial” AGI, which it defined in its founding charter as “highly autonomous systems that outperform humans at most economically valuable work”.
In a paper published in 2023, DeepMind researchers identified five ascending levels of AGI. They were (i) Emerging, “equal to or somewhat better than an unskilled human”; (ii) Competent, “at least 50th percentile of skilled adults”; (iii) Expert, at “least 90th percentile of skilled adults”; (iv) Virtuoso, “at least 99th percentile of skilled adults”; and (v) Superhuman, which “outperforms 100% of humans”.
The paper noted that in 2023, only the first level, Emerging AI, had been achieved.
Story continues below this ad
In his popular Machines of Loving Grace essay published in October 2024, Dario Amodei, CEO and co-founder of Anthropic, which is behind the LLM Claude, said he found AGI to be “an imprecise term that has gathered a lot of sci-fi baggage and hype”, and that he would “prefer ‘powerful AI’ or ‘Expert-Level Science and Engineering’”, which would mean the same “without the hype”.
There are also debates on the nature of intelligence itself.
Yann LeCun, Chief AI Scientist at Meta and a pioneer in the field of Deep Learning that lies at the heart of LLMs, has repeatedly said he does not agree with the term AGI, because “human intelligence is highly specialised”.
“Intelligence is a collection of skills and an ability to acquire new ones quickly. It cannot be measured with a scalar quantity. No intelligence can be even close to general, which is why the phrase ‘Artificial General Intelligence’ makes no sense,” LeCun, winner of the 2018 Turing Award, said early last year.
Story continues below this ad
“There is no question that machines will eventually equal and surpass human intelligence in all domains,” LeCun said. “But even those systems will not have ‘general’ intelligence, for any reasonable definition of the word general.”
According to LeCun, it won’t be possible to get to human-level AI simply by “scaling up” LLMs. In the next couple of years, there could perhaps be systems to help with “answers” that may feel like a “PhD is sitting next to you”. “But it is not a PhD… it is a system with gigantic memory and retrieval ability… But not a system that would invent solutions to new problems,” he said.
Bottomline: should we be worried about AGI, then?
According to Princeton University researchers Arvind Narayanan and Suyash Kapoor, a lot of researchers seem convinced that AGI “is an imminent existential threat requiring dramatic global action” (AI Snake Oil: What Artificial Intelligence Can Do, What It Can’t, and How to Tell the Difference, 2024).
Narayanan and Kapoor trace the history of AI’s evolution from the 1950s onward, and say that “at any given time, it is hard to tell whether the current dominant paradigm” in AI is the way to go or “if it is actually a dead end”.
Thus, the current path on LLMs could be one of many ways to reach AGI, or not be a way at all. We will start to figure things out with greater research and breakthroughs.
In the meantime, Narayanan and Kapoor argue, it is important to understand how this technology works, and assess its specific threats. This way we could frame sharper policies on how to interact with it — better than trying to frame it through an idea that has not yet been achieved.
Their conclusion: “AI is a general-purpose technology, and as such it will probably be of some help to those seeking to cause large-scale harm… If this creates added urgency to address civilisational threats, that’s a win. But reframing existing risks as AI risks would be a grave mistake, since trying to fix AI will have only a minimal impact on the real risks… As for the idea of rogue AI, that’s best left to the realm of science fiction.”
[ad_2]
Source link